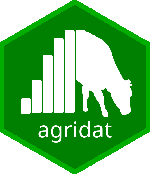
Yield monitor data from a corn field in Minnesota
gartner.corn.Rd
Yield monitor data from a corn field in Minnesota
Usage
data("gartner.corn")
Format
A data frame with 4949 observations on the following 8 variables.
long
longitude
lat
latitude
mass
grain mass flow per second, pounds
time
GPS time, in seconds
seconds
seconds elapsed for each datum
dist
distance traveled for each datum, in inches
moist
grain moisture, percent
elev
elevation, feet
Details
The data was collected 5 Nov 2011 from a corn field south of Mankato, Minnesota, using a combine-mounted yield monitor. https://www.google.com/maps/place/43.9237575,-93.9750632
Each harvested swath was 12 rows wide = 360 inches.
Timestamp 0 = 5 Nov 2011, 12:38:03 Central Time. Timestamp 16359 = 4.54 hours later.
Yield is calculated as total dry weight (corrected to 15.5 percent moisture), divided by 56 pounds (to get bushels), divided by the harvested area:
drygrain = [massflow * seconds * (100-moisture) / (100-15.5)] / 56 harvested area = (distance * swath width) / 6272640 yield = drygrain / area
Source
University of Minnesota Precision Agriculture Center. Retrieved 27 Aug 2015 from https://web.archive.org/web/20100717003256/https://www.soils.umn.edu/academics/classes/soil4111/files/yield_a.xls
Used via license: Creative Commons BY-SA 3.0.
References
Suman Rakshit, Adrian Baddeley, Katia Stefanova, Karyn Reeves, Kefei Chen, Zhanglong Cao, Fiona Evans, Mark Gibberd (2020). Novel approach to the analysis of spatially-varying treatment effects in on-farm experiments. Field Crops Research, 255, 15 September 2020, 107783. https://doi.org/10.1016/j.fcr.2020.107783
Examples
if (FALSE) { # \dontrun{
library(agridat)
data(gartner.corn)
dat <- gartner.corn
# Calculate yield from mass & moisture
dat <- transform(dat,
yield=(mass*seconds*(100-moist)/(100-15.5)/56)/(dist*360/6272640))
# Delete low yield outliers
dat <- subset(dat, yield >50)
# Group yield into 20 bins for red-gray-blue colors
medy <- median(dat$yield)
ncols <- 20
wwidth <- 150
brks <- seq(from = -wwidth/2, to=wwidth/2, length=ncols-1)
brks <- c(-250, brks, 250) # 250 is safe..we cleaned data outside ?(50,450)?
yldbrks <- brks + medy
dat <- transform(dat, yldbin = as.numeric(cut(yield, breaks= yldbrks)))
redblue <- colorRampPalette(c("firebrick", "lightgray", "#375997"))
dat$yieldcolor = redblue(ncols)[dat$yldbin]
# Polygons for soil map units
# Go to: https://websoilsurvey.nrcs.usda.gov/app/WebSoilSurvey.aspx
# Click: Lat and Long. 43.924, -93.975
# Click the little AOI rectangle icon. Drag around the field
# In the AOI Properties, enter the Name: Gartner
# Click the tab Soil Map to see map unit symbols, names
# Click: Download Soils Data. Click: Create Download Link.
# Download the zip file and find the soilmu_a_aoi files.
# Read shape files
libs(sf)
fname <- system.file(package="agridat", "files", "gartner.corn.shp")
shp <- sf::st_read( fname )
# Annotate soil map units. Coordinates chosen by hand.
mulabs = data.frame(
name=c("110","319","319","230","105C","110","211","110","211","230","105C"),
x = c(-93.97641, -93.97787, -93.97550, -93.97693, -93.97654, -93.97480,
-93.97375, -93.978284, -93.977617, -93.976715, -93.975929),
y = c(43.92185, 43.92290, 43.92358, 43.92445, 43.92532, 43.92553,
43.92568, 43.922163, 43.926427, 43.926993, 43.926631) )
mulabs = st_as_sf( mulabs, coords=c("x","y"), crs=4326)
mulabs = st_transform(mulabs, 2264)
# Trim top and bottom ends of the field
dat <- subset(dat, lat < 43.925850 & lat > 43.921178)
# Colored points for yield
dat <- st_as_sf(dat, coords=c("long","lat"), crs=4326)
libs(ggplot2)
ggplot() +
geom_sf(data=dat, aes(col=yieldcolor) ) +
scale_color_identity() +
geom_sf_label(data=mulabs, aes(label=name), cex=2) +
geom_sf(data=shp["MUSYM"], fill="transparent") +
ggtitle("gartner.corn") +
theme_classic()
if(0){
# Draw a 3D surface. Clearly shows the low drainage area
# Re-run the steps above up, stop before the "Colored points" line.
libs(rgl)
dat <- transform(dat, x=long-min(long), y=lat-min(lat), z=elev-min(elev))
clear3d()
points3d(dat$x, dat$y, dat$z/50000,
col=redblue(ncols)[dat$yldbin])
axes3d()
title3d(xlab='x',ylab='y',zlab='elev')
close3d()
}
} # }