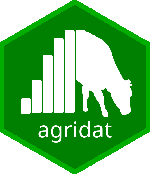
Wireworms controlled by fumigants in a latin square
cochran.wireworms.Rd
Wireworms controlled by fumigants in a latin square
Format
A data frame with 25 observations on the following 4 variables.
row
row
col
column
trt
fumigant treatment, 5 levels
worms
count of wireworms per plot
Details
Plots were approximately 22 cm by 13 cm. Layout of the experiment was a latin square. The number of wireworms in each plot was counted, following soil fumigation the previous year.
Source
W. G. Cochran (1938). Some difficulties in the statistical analysis of replicated experiments. Empire Journal of Experimental Agriculture, 6, 157–175.
References
Ron Snee (1980). Graphical Display of Means. The American Statistician, 34, 195-199. https://www.jstor.org/stable/2684060 https://doi.org/10.1080/00031305.1980.10483028
W. Cochran (1940). The analysis of variance when experimental errors follow the Poisson or binomial laws. The Annals of Mathematical Statistics, 11, 335-347. https://www.jstor.org/stable/2235680
G W Snedecor and W G Cochran, 1980. Statistical Methods, Iowa State University Press. Page 288.
Examples
if (FALSE) { # \dontrun{
library(agridat)
data(cochran.wireworms)
dat <- cochran.wireworms
libs(desplot)
desplot(dat, worms ~ col*row,
text=trt, cex=1, # aspect unknown
main="cochran.wireworms")
# Trt K is effective, but not the others. Really, this says it all.
libs(lattice)
bwplot(worms ~ trt, dat, main="cochran.wireworms", xlab="Treatment")
# Snedecor and Cochran do ANOVA on sqrt(x+1).
dat <- transform(dat, rowf=factor(row), colf=factor(col))
m1 <- aov(sqrt(worms+1) ~ rowf + colf + trt, data=dat)
anova(m1)
# Instead of transforming, use glm
m2 <- glm(worms ~ trt + rowf + colf, data=dat, family="poisson")
anova(m2)
# GLM with random blocking.
libs(lme4)
m3 <- glmer(worms ~ -1 +trt +(1|rowf) +(1|colf), data=dat, family="poisson")
summary(m3)
## Fixed effects:
## Estimate Std. Error z value Pr(>|z|)
## trtK 0.1393 0.4275 0.326 0.745
## trtM 1.7814 0.2226 8.002 1.22e-15 ***
## trtN 1.9028 0.2142 8.881 < 2e-16 ***
## trtO 1.7147 0.2275 7.537 4.80e-14 ***
} # }