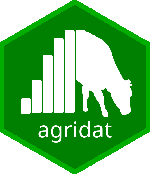
Cucumber yields and quantitative traits
cramer.cucumber.Rd
Cucumber yields and quantitative traits
Usage
data("cramer.cucumber")
Format
A data frame with 24 observations on the following 9 variables.
cycle
cycle
rep
replicate
plants
plants per plot
flowers
number of pistillate flowers
branches
number of branches
leaves
number of leaves
totalfruit
total fruit number
culledfruit
culled fruit number
earlyfruit
early fruit number
Details
The data are used to illustrate path analysis of the correlations between phenotypic traits.
Used with permission of Christopher Cramer.
Source
Christopher S. Cramer, Todd C. Wehner, and Sandra B. Donaghy. 1999. Path Coefficient Analysis of Quantitative Traits. In: Handbook of Formulas and Software for Plant Geneticists and Breeders, page 89.
References
Cramer, C. S., T. C. Wehner, and S. B. Donaghy. 1999. PATHSAS: a SAS computer program for path coefficient analysis of quantitative data. J. Hered, 90, 260-262 https://doi.org/10.1093/jhered/90.1.260
Examples
if (FALSE) { # \dontrun{
library(agridat)
data(cramer.cucumber)
dat <- cramer.cucumber
libs(lattice)
splom(dat[3:9], group=dat$cycle,
main="cramer.cucumber - traits by cycle",
auto.key=list(columns=3))
# derived traits
dat <- transform(dat,
marketable = totalfruit-culledfruit,
branchesperplant = branches/plants,
nodesperbranch = leaves/(branches+plants),
femalenodes = flowers+totalfruit)
dat <- transform(dat,
perfenod = (femalenodes/leaves),
fruitset = totalfruit/flowers,
fruitperplant = totalfruit / plants,
marketableperplant = marketable/plants,
earlyperplant=earlyfruit/plants)
# just use cycle 1
dat1 <- subset(dat, cycle==1)
# define independent and dependent variables
indep <- c("branchesperplant", "nodesperbranch", "perfenod", "fruitset")
dep0 <- "fruitperplant"
dep <- c("marketable","earlyperplant")
# standardize trait data for cycle 1
sdat <- data.frame(scale(dat1[1:8, c(indep,dep0,dep)]))
# slopes for dep0 ~ indep
X <- as.matrix(sdat[,indep])
Y <- as.matrix(sdat[,c(dep0)])
# estdep <- solve(t(X)
estdep <- solve(crossprod(X), crossprod(X,Y))
estdep
## branchesperplant 0.7160269
## nodesperbranch 0.3415537
## perfenod 0.2316693
## fruitset 0.2985557
# slopes for dep ~ dep0
X <- as.matrix(sdat[,dep0])
Y <- as.matrix(sdat[,c(dep)])
# estind2 <- solve(t(X)
estind2 <- solve(crossprod(X), crossprod(X,Y))
estind2
## marketable earlyperplant
## 0.97196 0.8828393
# correlation coefficients for indep variables
corrind=cor(sdat[,indep])
round(corrind,2)
## branchesperplant nodesperbranch perfenod fruitset
## branchesperplant 1.00 0.52 -0.24 0.09
## nodesperbranch 0.52 1.00 -0.44 0.14
## perfenod -0.24 -0.44 1.00 0.04
## fruitset 0.09 0.14 0.04 1.00
# Correlation coefficients for dependent variables
corrdep=cor(sdat[,c(dep0, dep)])
round(corrdep,2)
## fruitperplant marketable earlyperplant
## fruitperplant 1.00 0.97 0.88
## marketable 0.97 1.00 0.96
## earlyperplant 0.88 0.96 1.00
result = corrind
result = result*matrix(estdep,ncol=4,nrow=4,byrow=TRUE)
round(result,2) # match SAS output columns 1-4
## branchesperplant nodesperbranch perfenod fruitset
## branchesperplant 0.72 0.18 -0.06 0.03
## nodesperbranch 0.37 0.34 -0.10 0.04
## perfenod -0.17 -0.15 0.23 0.01
## fruitset 0.07 0.05 0.01 0.30
resdep0 = rowSums(result)
resdep <- cbind(resdep0,resdep0)*matrix(estind2, nrow=4,ncol=2,byrow=TRUE)
colnames(resdep) <- dep
# slightly different from SAS output last 2 columns
round(cbind(fruitperplant=resdep0, round(resdep,2)),2)
## fruitperplant marketable earlyperplant
## branchesperplant 0.87 0.84 0.76
## nodesperbranch 0.65 0.63 0.58
## perfenod -0.08 -0.08 -0.07
## fruitset 0.42 0.41 0.37
} # }