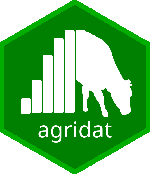
Yield of corn, alfalfa, clover with two fertilizers
heady.fertilizer.Rd
Yield of corn, alfalfa, clover with two fertilizers
Usage
data("heady.fertilizer")
Format
A data frame with 81 observations on the following 3 variables.
crop
crop
rep
replicate (not block)
P
phosphorous, pounds/acre
K
potassium, pounds/acre
N
nitrogen, pounds/acre
yield
yield
Details
Heady et al. fit two-variable semi-polynomial response surfaces for each crop.
Clover and alfalfa yields are in tons/acre. The clover and alfalfa experiments were grown in 1952.
Corn yields are given as bu/acre. The corn experiments were grown in 1952 and 1953. The same test plots were used in 1953 and in 1952, but no fertilizer was applied in 1953–any response in yield is due to residual fertilizer from 1952.
All experiments used an incomplete factorial design. Not all treatment combinations were present.
Source
Earl O. Heady, John T. Pesek, William G. Brown. (1955). Crop Response Surfaces and Economic Optima in Fertilizer Use. Agricultural Experiment Station, Iowa State College. Research bulletin 424. Pages 330-332. https://lib.dr.iastate.edu/cgi/viewcontent.cgi?filename=12&article=1032&context=ag_researchbulletins&type=additional
References
Pesek, John and Heady, Earl O. 1956. A two nutrient-response function with determination of economic optima for the rate and grade of fertilizer for alfalfa. Soil Science Society of America Journal, 20, 240-246. https://doi.org/10.2136/sssaj1956.03615995002000020025x
Examples
if (FALSE) { # \dontrun{
library(agridat)
data(heady.fertilizer)
dat <- heady.fertilizer
libs(lattice)
xyplot(yield ~ P|crop, data=dat, scales=list(relation="free"),
groups=factor(paste(dat$N,dat$K)), auto.key=list(columns=5),
main="heady.fertilizer", xlab="Phosphorous")
# Corn. Matches Heady, p. 292
d1 <- subset(dat, crop=="corn")
m1 <- lm(yield ~ N + P + sqrt(N) + sqrt(P) + sqrt(N*P), data=d1)
summary(m1)
# Alfalfa. Matches Heady, p. 292. Also Pesek equation 3, p. 241
d2 <- subset(dat, crop=="alfalfa")
m2 <- lm(yield ~ K + P + sqrt(K) + sqrt(P) + sqrt(K*P), data=d2)
summary(m2)
## Coefficients:
## Estimate Std. Error t value Pr(>|t|)
## (Intercept) 1.8735521 0.1222501 15.326 < 2e-16 ***
## K -0.0013943 0.0007371 -1.891 0.061237 .
## P -0.0050195 0.0007371 -6.810 5.74e-10 ***
## sqrt(K) 0.0617458 0.0160142 3.856 0.000196 ***
## sqrt(P) 0.1735383 0.0160142 10.837 < 2e-16 ***
## sqrt(K * P) -0.0014402 0.0007109 -2.026 0.045237 *
# Clover. Matches Heady, p. 292.
d3 <- subset(dat, crop=="clover")
m3 <- lm(yield ~ P + sqrt(K) + sqrt(P) + sqrt(K*P), data=d3)
summary(m3)
# Corn with residual fertilizer. Matches Heady eq 56, p. 322.
d4 <- subset(dat, crop=="corn2")
m4 <- lm(yield ~ N + P + sqrt(N) + sqrt(P) + sqrt(N*P), data=d4)
summary(m4)
libs(rgl)
with(d1, plot3d(N,P,yield))
with(d2, plot3d(K,P,yield))
with(d3, plot3d(K,P,yield))
with(d4, plot3d(N,P,yield)) # Mostly linear in both N and P
close3d()
} # }