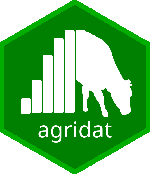
Alpha lattice design of spring oats
john.alpha.Rd
Alpha lattice design of spring oats
Format
A data frame with 72 observations on the following 5 variables.
plot
plot number
rep
replicate
block
incomplete block
gen
genotype (variety)
yield
dry matter yield (tonnes/ha)
row
Row ordinate
col
Column ordinate
Details
A spring oats trial grown in Craibstone, near Aberdeen. There were 24 varieties in 3 replicates, each consisting of 6 incomplete blocks of 4 plots. Planted in a resolvable alpha design.
Caution: Note that the table on page 146 of John & Williams (1995) is NOT the physical layout. The plots were laid out in a single line.
Source
J. A. John & E. R. Williams (1995). Cyclic and computer generated designs. Chapman and Hall, London. Page 146.
References
Piepho, H.P. and Mohring, J. (2007), Computing heritability and selection response from unbalanced plant breeding trials. Genetics, 177, 1881-1888. https://doi.org/10.1534/genetics.107.074229
Paul Schmidt, Jens Hartung, Jörn Bennewitz, and Hans-Peter Piepho (2019). Heritability in Plant Breeding on a Genotype-Difference Basis. Genetics, 212, 991-1008. https://doi.org/10.1534/genetics.119.302134
Examples
if (FALSE) { # \dontrun{
library(agridat)
data(john.alpha)
dat <- john.alpha
# RCB (no incomplete block)
m0 <- lm(yield ~ 0 + gen + rep, data=dat)
# Block fixed (intra-block analysis) (bottom of table 7.4 in John)
m1 <- lm(yield ~ 0 + gen + rep + rep:block, dat)
anova(m1)
# Block random (combined inter-intra block analysis)
libs(lme4, lucid)
m2 <- lmer(yield ~ 0 + gen + rep + (1|rep:block), dat)
anova(m2)
## Analysis of Variance Table
## Df Sum Sq Mean Sq F value
## gen 24 380.43 15.8513 185.9942
## rep 2 1.57 0.7851 9.2123
vc(m2)
## grp var1 var2 vcov sdcor
## rep:block (Intercept) <NA> 0.06194 0.2489
## Residual <NA> <NA> 0.08523 0.2919
# Variety means. John and Williams table 7.5. Slight, constant
# difference for each method as compared to John and Williams.
means <- data.frame(rcb=coef(m0)[1:24],
ib=coef(m1)[1:24],
intra=fixef(m2)[1:24])
head(means)
## rcb ib intra
## genG01 5.201233 5.268742 5.146433
## genG02 4.552933 4.665389 4.517265
## genG03 3.381800 3.803790 3.537934
## genG04 4.439400 4.728175 4.528828
## genG05 5.103100 5.225708 5.075944
## genG06 4.749067 4.618234 4.575394
libs(lattice)
splom(means, main="john.alpha - means for RCB, IB, Intra-block")
# ----------
if(require("asreml", quietly=TRUE)){
libs(asreml,lucid)
# Heritability calculation of Piepho & Mohring, Example 1
m3 <- asreml(yield ~ 1 + rep, data=dat, random=~ gen + rep:block)
sg2 <- summary(m3)$varcomp['gen','component'] # .142902
# Average variance of a difference of two adjusted means (BLUP)
p3 <- predict(m3, data=dat, classify="gen", sed=TRUE)
# Matrix of pair-wise SED values, squared
vdiff <- p3$sed^2
# Average variance of two DIFFERENT means (using lower triangular of vdiff)
vblup <- mean(vdiff[lower.tri(vdiff)]) # .05455038
# Note that without sed=TRUE, asreml reports square root of the average variance
# of a difference between the variety means, so the following gives the same value
# predict(m3, data=dat, classify="gen")$avsed ^ 2 # .05455038
# Average variance of a difference of two adjusted means (BLUE)
m4 <- asreml(yield ~ 1 + gen + rep, data=dat, random = ~ rep:block)
p4 <- predict(m4, data=dat, classify="gen", sed=TRUE)
vdiff <- p4$sed^2
vblue <- mean(vdiff[lower.tri(vdiff)]) # .07010875
# Again, could use predict(m4, data=dat, classify="gen")$avsed ^ 2
# H^2 Ad-hoc measure of heritability
sg2 / (sg2 + vblue/2) # .803
# H^2c Similar measure proposed by Cullis.
1-(vblup / (2*sg2)) # .809
}
# ----------
# lme4 to calculate Cullis H2
# https://stackoverflow.com/questions/38697477
libs(lme4)
cov2sed <- function(x){
# Convert var-cov matrix to SED matrix
# sed[i,j] = sqrt( x[i,i] + x[j,j]- 2*x[i,j] )
n <- nrow(x)
vars <- diag(x)
sed <- sqrt( matrix(vars, n, n, byrow=TRUE) +
matrix(vars, n, n, byrow=FALSE) - 2*x )
diag(sed) <- 0
return(sed)
}
# Same as asreml model m4. Note 'gen' must be first term
m5blue <- lmer(yield ~ 0 + gen + rep + (1|rep:block), dat)
libs(emmeans)
ls5blue <- emmeans(m5blue, "gen")
con <- ls5blue@linfct[,1:24] # contrast matrix for genotypes
# The 'con' matrix is identity diagonal, so we don't need to multiply,
# but do so for a generic approach
# sed5blue <- cov2sed(con
tmp <- tcrossprod( crossprod(t(con), vcov(m5blue)[1:24,1:24]), con)
sed5blue <- cov2sed(tmp)
# vblue Average variance of difference between genotypes
vblue <- mean(sed5blue[upper.tri(sed5blue)]^2)
vblue # .07010875 matches 'vblue' from asreml
# Now blups
m5blup <- lmer(yield ~ 0 + (1|gen) + rep + (1|rep:block), dat)
# Need lme4::ranef in case ordinal is loaded
re5 <- lme4::ranef(m5blup,condVar=TRUE)
vv1 <- attr(re5$gen,"postVar")
vblup <- 2*mean(vv1) # .0577 not exactly same as 'vblup' above
vblup
# H^2 Ad-hoc measure of heritability
sg2 <- c(lme4::VarCorr(m5blup)[["gen"]]) # 0.142902
sg2 / (sg2 + vblue/2) # .803 matches asreml
# H^2c Similar measure proposed by Cullis.
1-(vblup / 2 / sg2) # .809 from asreml, .800 from lme4
# ----------
# Sommer to calculate Cullis H2
libs(sommer)
m2.ran <- mmer(fixed = yield ~ rep,
random = ~ gen + rep:block,
data = dat)
vc_g <- m2.ran$sigma$gen # genetic variance component
n_g <- n_distinct(dat$gen) # number of genotypes
C22_g <- m2.ran$PevU$gen$yield # Prediction error variance matrix for genotypic BLUPs
trC22_g <- sum(diag(C22_g)) # trace
# Mean variance of a difference between genotypic BLUPs. Smith eqn 26
# I do not see the algebraic reason for this...2
av2 <- 2/n_g * (trC22_g - (sum(C22_g)-trC22_g) / (n_g-1))
### H2 Cullis
1-(av2 / (2 * vc_g)) #0.8091
} # }