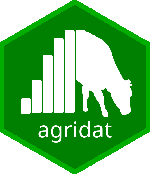
Uniformity trial of barley
kempton.barley.uniformity.Rd
Uniformity trial of barley at Cambridge, England, 1978.
Format
A data frame with 196 observations on the following 3 variables.
row
row
col
column
yield
grain yield, kg
Details
A uniformity trial of spring barley planted in 1978. Conducted by the Plant Breeding Institute in Cambridge, England.
Each plot is 5 feet wide, 14 feet long.
Field width: 7 plots * 14 feet = 98 feet
Field length: 28 plots * 5 feet = 140 feet
Source
R. A. Kempton and C. W. Howes (1981). The use of neighbouring plot values in the analysis of variety trials. Applied Statistics, 30, 59–70. https://doi.org/10.2307/2346657
References
McCullagh, P. and Clifford, D., (2006). Evidence for conformal invariance of crop yields, Proceedings of the Royal Society A: Mathematical, Physical and Engineering Science. 462, 2119–2143. https://doi.org/10.1098/rspa.2006.1667
Examples
if (FALSE) { # \dontrun{
library(agridat)
data(kempton.barley.uniformity)
dat <- kempton.barley.uniformity
libs(desplot)
desplot(dat, yield~col*row,
aspect=140/98, tick=TRUE, # true aspect
main="kempton.barley.uniformity")
# Kempton estimated auto-regression coefficients b1=0.10, b2=0.91
dat <- transform(dat, xf = factor(col), yf=factor(row))
# ----------
if(require("asreml", quietly=TRUE)){
libs(asreml,lucid)
dat <- transform(dat, xf = factor(col), yf=factor(row))
m1 <- asreml(yield ~ 1, data=dat, resid = ~ar1(xf):ar1(yf))
# lucid::vc(m1)
## effect component std.error z.ratio bound
## xf:yf!R 0.1044 0.02197 4.7 P 0
## xf:yf!xf!cor 0.2458 0.07484 3.3 U 0
## xf:yf!yf!cor 0.8186 0.03821 21 U 0
# asreml estimates auto-regression correlations of 0.25, 0.82
# Kempton estimated auto-regression coefficients b1=0.10, b2=0.91
}
# ----------
if(0){
# Kempton defines 4 blocks, randomly assigns variety codes 1-49 in each block, fits
# RCB model, computes mean squares for variety and residual. Repeat 40 times.
# Kempton's estimate: variety = 1032, residual = 1013
# Our estimate: variety = 825, residual = 1080
fitfun <- function(dat){
dat <- transform(dat, block=factor(ceiling(row/7)),
gen=factor(c(sample(1:49),sample(1:49),sample(1:49),sample(1:49))))
m2 <- lm(yield*100 ~ block + gen, dat)
anova(m2)[2:3,'Mean Sq']
}
set.seed(251)
out <- replicate(50, fitfun(dat))
rowMeans(out) # 826 1079
}
} # }