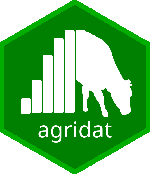
Multi-environment trial of wheat cultivars introduced 1860-1982.
perry.springwheat.Rd
Yields of wheat cultivars introduced 1860-1982. Grown in 20 environments.
Usage
data("perry.springwheat")
Format
A data frame with 560 observations on the following 6 variables.
yield
yield, kg/ha
gen
genotype/cultivar factor, 28 levels
env
environment factor, 20 levels
site
site factor
year
year, 1979-1982
yor
year of release, 1860-1982
Details
Twenty-eight of the most significant wheat cultivars of the past century in Western Australia, were grown in 20 field trials over 4 years in the Central and Eastern wheat-belt of Australia.
At the Wongan Hills site there were separate early and late sown trials in 1979 and 1980. Later sowing dates generally have lower yields.
Note: Although not indicated by the original paper, it may be that the Merredin site in 1979 also had early/late sowing dates.
Used with permission of Mario D'Antuono and CSIRO Publishing.
Source
MW Perry and MF D'Antuono. (1989). Yield improvement and associated characteristics of some Australian spring wheat cultivars introduced between 1860 and 1982. Australian Journal of Agricultural Research, 40(3), 457–472. https://www.publish.csiro.au/nid/43/issue/1237.htm
Examples
if (FALSE) { # \dontrun{
library(agridat)
data(perry.springwheat)
dat <- perry.springwheat
libs(lattice)
xyplot(yield~yor|env, dat, type=c('p','r'), xlab="year of release",
main="perry.springwheat")
# Show the genetic trend for each testing location * year.
# libs(latticeExtra)
# useOuterStrips(xyplot(yield~yor|site*factor(year), dat,
# type=c('p','r')))
# Perry reports a rate of gain of 5.8 kg/ha/year. No model is given.
# We fit a model with separate intercept/slope for each env
m1 <- lm(yield ~ env + yor + env:yor, data=dat)
# Average slope across environments
mean(c(coef(m1)[21], coef(m1)[21]+coef(m1)[22:40]))
## [1] 5.496781
# ----------
# Now a mixed-effects model. Fixed overall int/slope. Random env int/slope.
# First, re-scale response so we don't have huge variances
dat$y <- dat$yield / 100
libs(lme4)
# Use || for uncorrelated int/slope. Bad model. See below.
# m2 <- lmer(y ~ 1 + yor + (1+yor||env), data=dat)
## Warning messages:
## 1: In checkConv(attr(opt, "derivs"), opt$par, ctrl = control$checkConv, :
## Model failed to converge with max|grad| = 0.55842 (tol = 0.002, component 1)
## 2: In checkConv(attr(opt, "derivs"), opt$par, ctrl = control$checkConv, :
## Model is nearly unidentifiable: very large eigenvalue
## - Rescale variables?;Model is nearly unidentifiable: large eigenvalue ratio
## - Rescale variables?
# Looks like lme4 is having trouble with variance of intercepts
# There is nothing special about 1800 years, so change the
# intercept -- 'correct' yor by subtracting 1800 and try again.
dat$yorc <- dat$yor - 1800
m3 <- lmer(y ~ 1 + yorc + (1+yorc||env), data=dat)
# Now lme4 succeeds. Rate of gain is 100*0.0549 = 5.49
fixef(m3)
## (Intercept) yorc
## 5.87492444 0.05494464
if(require("asreml", quietly=TRUE)){
libs(asreml,lucid)
m3a <- asreml(y ~ 1 + yorc, data=dat, random = ~ env + env:yorc)
lucid::vc(m3)
## grp var1 var2 vcov sdcor
## env (Intercept) <NA> 11.61 3.407
## env.1 yorc <NA> 0.00063 0.02511
## Residual <NA> <NA> 3.551 1.884
lucid::vc(m3a)
## effect component std.error z.ratio con
## env!env.var 11.61 4.385 2.6 Positive
## env:yorc!env.var 0.00063 0.000236 2.7 Positive
## R!variance 3.551 0.2231 16 Positive
}
} # }